In the world of identity management and security, fraudsters are constantly evolving their tactics, exploiting weaknesses in outdated device identification methods. For fraud, identity and digital experience teams, securing device identity has never been more crucial. However, many organizations still rely on fragmented, outdated solutions that are no longer effective.
A tale of trust and security
Now imagine a user named Sarah. She’s just logged into her banking app, eager to check her balance before making a significant purchase. But unbeknownst to her, fraudsters are watching, ready to exploit any weaknesses in the bank’s security measures.
In this case, Sarah’s bank relied on traditional device identification methods to verify her device and, unfortunately, fraudsters exploited these weaknesses. By hijacking cookies from a previous session, they were able to mimic Sarah’s device, making it look like the transaction request was coming from her phone. At the same time, mobile device IDs were spoofed, and no alarms were raised.
In this blog post, part of our ongoing Identity-Based Fraud Prevention blog series, we explore the limitations of traditional device identification methods and emphasize the importance of adopting a multi-layered strategy. By blending explicit identity mechanisms with implicit signals — like behavioral biometrics — we can create a safer, more secure digital experience for users like Sarah.
Traditional device identification: Where it falls short
Legacy systems often rely on methods like cookies, tokens and mobile OS-reported device IDs. While these techniques have been widely used, they come with serious limitations:
- Cookies and tokens: Easily stolen and replayed, allowing attackers to hijack sessions or impersonate legitimate users.
- Mobile device IDs: Though somewhat reliable, these can be erased or spoofed, which diminishes their effectiveness.
- Browser fingerprinting: Limited by privacy regulations that restrict data collection, rendering this method less effective.
As Sarah’s story illustrates, these traditional methods are becoming obsolete. The potential deprecation of cookies and the rise of sophisticated fraud tactics demand a more robust, adaptable solution.
The importance of persistent identity
Traditionally, organizations have had two main approaches to device identification: explicit and implicit identity. Different vendors and identity providers have tried to improve their security measures by offering one or the other. However, focusing on just one of these methods often leaves gaps that fraudsters can exploit, as neither approach is foolproof on its own.
- Explicit identity involves concrete identifiers actively provided by the device, such as crypto-binding keys. Think of this as using a credit card with a chip — much more secure and difficult for attackers to bypass.
- Implicit identity gathers signals passively from device characteristics or behaviors without active declarations. While essential for fraud detection, these signals serve more as supporting evidence, similar to circumstantial clues at a crime scene.
To ensure the best security for users like Sarah, organizations should start relying on persistent explicit identity, using implicit signals to detect anomalies and validate device trustworthiness. This layered approach not only strengthens security but also minimizes the risk of false positives.
Why a multi-layered approach is essential
Fraud tactics are constantly evolving; a static approach to device identification simply isn’t enough. A multi-layered approach that incorporates multiple types of signals ensures better coverage and resilience against fraud. Key components of this strategy include:
- Crypto-binding keys: These cryptographic anchors are tied to the device’s hardware, offering a secure, persistent form of identification. While they can be erased by the user who owns the device, they can’t be accessed or stolen by attackers. The device must sign a cryptographic challenge, which is then verified by the system—similar to how a physical chip on a credit card is verified by a payment terminal.
- Device fingerprinting: By collecting and analyzing hundreds of characteristics, device fingerprinting creates a dynamic profile of the device, allowing for the detection of subtle changes and potential spoofing attempts.
- Behavioral biometrics: Analyzing how users interact with their devices — typing patterns, swiping behavior — adds another layer of protection to detect fraud.
- AI-driven behavioral profiling: Incorporating User and Entity Behavior Analytics (UEBA) powered by AI can significantly enhance fraud detection by continuously profiling users and understanding their context.
- Mobile-native security: As mobile apps become primary targets for fraudsters, securing mobile devices is essential for protecting both users and businesses. Mobile-native security addresses threats like malware, remote access control and overlay attacks, which exploit already trusted devices. For instance, fraudsters may use malware to monitor device activity or launch overlay attacks to mimic legitimate app interfaces, tricking users into providing sensitive information. By detecting and mitigating these types of threats, mobile-native security ensures that even trusted devices remain protected against sophisticated exploitation attempts.
The power of collaboration
In the evolving world of fraud prevention, collaboration is becoming a powerful tool. By sharing passive telemetry data — such as device fingerprints and organized behavioral patterns — alongside risk indicators and threat intelligence, a consortium of organizations, banks and businesses can collectively build stronger defenses. This shared intelligence enables them to detect suspicious activity earlier, allowing swift action before fraud becomes widespread.
When this collaborative approach is combined with a layered strategy of explicit and implicit identity signals, it can significantly improve the accuracy of device identification and help mitigate known risks. For instance, in Sarah’s case, the bank could detect suspicious activity from reused cookies — a common credential-stuffing tactic — and take proactive measures to protect her account. Rather than allowing access, the bank’s system could require step-up authentication, rejecting the fraud attempt and alerting Sarah to the risk. This message could encourage her to register a strong authentication method, such as passkeys, further safeguarding her account from future attacks that rely on compromised credentials. This layered, proactive approach empowers users like Sarah to strengthen their own security while enhancing the bank’s defenses against advanced threats.
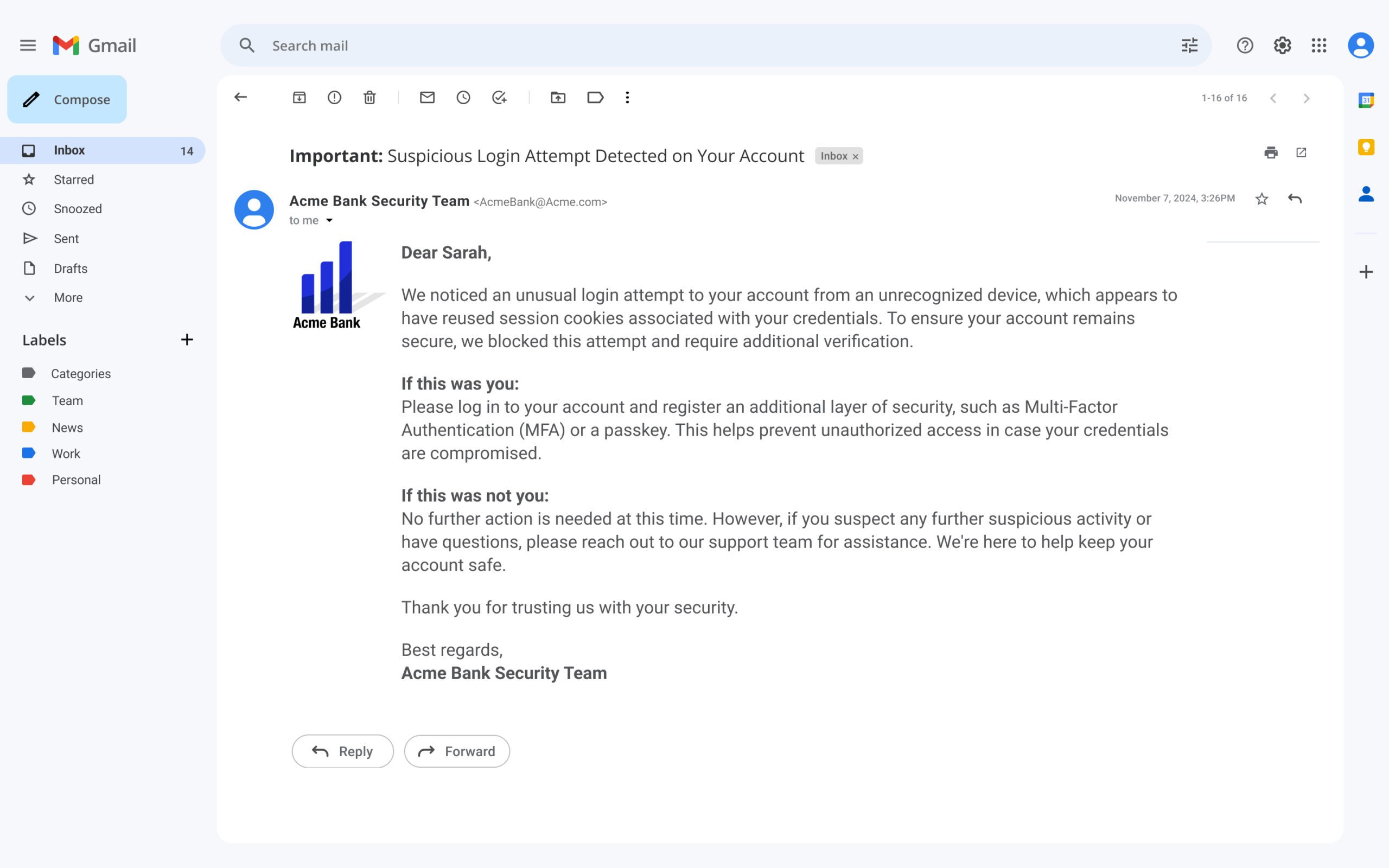
Moreover, this layered approach allows the bank to not only establish trust with Sarah but also empower her to set her own trust thresholds. Whether Sarah prefers stricter device checks or a more frictionless experience, the bank could tailor its security measures to her comfort level. This flexibility ensures a balance between security and user experience, without leaving any cracks for fraudsters to exploit.
By leveraging collaboration and adopting a multi-layered strategy, organizations can keep customers like Sarah safe while enhancing their overall experience. The result is a system that continuously adapts to emerging fraud tactics, ensuring that security evolves alongside convenience.
Conclusion: A layered approach is the future of device intelligence
As fraud prevention requires a multi-faceted, layered approach to device identification, organizations must adapt to evolving tactics and shifting consumer behaviors. To build a secure and frictionless digital experience, businesses should combine:
- Crypto-binding keys for strong, persistent device identity.
- Device fingerprinting to track changes, detect anomalies and identify anonymized traffic.
- Behavioral biometrics and AI-driven profiling for continuous trust evaluation.
- Mobile-native security to address the growing mobile fraud threat and protect mobile endpoints/devices.
By embracing this layered strategy that prioritizes both explicit and implicit identity signals, businesses can enhance their fraud detection capabilities, secure their users and stay one step ahead of fraudsters. This approach not only protects the bottom line but also fosters a seamless and positive user experience — encouraging customers like Sarah to stay loyal and expand their engagement, rather than seeking more reliable alternatives elsewhere.