Device fingerprinting capabilities should be considered a key capability for any fraud detection service. But as fraudsters continue to develop new and sophisticated methods of evading detection, even the most robust fingerprinting must be combined with additional detection and response methods to distinguish trusted users from attackers and block fraud tactics that can be used to compromise trusted devices or bypass device reputation measures.
In this follow-up blog post to our first post on device fingerprinting, we’ll explain some of the key challenges with creating strong device fingerprints, how fraudsters evade device reputation measures and the detection methods used on the Transmit Security Platform to create robust fingerprints and supplement them with additional detection methods.
Challenges with creating robust device fingerprints
Because device fingerprints are based on unique hardware and software properties that can change over time, may contain similarities to other devices with similar settings and can be masked by both legitimate and malicious users, fingerprints must be constantly updated to ensure a sufficient level of accuracy.
Some of the challenges associated with device fingerprints include:
- Increased privacy measures designed to give users more control over targeted advertisements and marketing tactics that leverage fingerprints
- Asynchronous updates to OS, devices, and browsers that change the properties used to identify devices
- Legitimate users who use incognito browsers, multiple devices or change devices due to upgrades, theft or loss
- Known and evolving device manipulations used by sophisticated fraudsters to evade device detection
Especially with regard to fraud evasion tactics, a constantly changing landscape — including generative AI capabilities that are making it easier than ever to mount sophisticated attacks easier — overcoming these challenges becomes not only difficult, but impossible to do without additional detection capabilities.
Can fraudsters evade robust device fingerprints?
While leveraging robust device fingerprints gives security and fraud teams a powerful tool against fraudsters, an increase in organized crime and an ever-evolving range of fraud tactics can enable fraudsters to bypass device reputation checks and evade even strong fingerprints.
Some of the advanced techniques used to evade robust device fingerprints include:
- Device takeover and remote access: Device takeover is an attack where hackers gain remote access to the victim’s device, typically by exploiting a remote desktop connection (RDC). By hacking into users’ devices, attackers gain unauthorized access to the victim’s online accounts (such as email or bank accounts) to commit ATO and other malicious activities.
- Advanced scams and social engineering: Advanced social engineering can trick users into sending money or assets to a fraudster posing as a genuine payee. This means the fraudulent activity occurs on the user’s device, effectively bypassing device fingerprinting protections. And as generative AI provides out-of-the-box tools for voice cloning that can trick users into believing they are interacting with trusted contacts, this threat only continues to grow.
- Device spoofing: Device spoofing is a technique where fraudsters manipulate various aspects of their device or system settings to fool you into thinking they’re legitimate. This way, fraudsters can create infinite devices and pass the device’s fingerprint reputation. Legitimate tools like OpenBullet 2.0, which are increasingly exploited to automate and scale these sophisticated attacks, provide fraudsters with the ability to make these attacks even harder to detect.
- Emulators: A mobile emulator is a software that mimics the hardware and software functionalities of smartphones. Although they have legitimate uses, such as developing and testing mobile applications or replicating the experience of using different gaming consoles, cybercriminals increasingly use emulators to bypass security measures by masquerading as legitimate and trusted devices. This way, fraudsters can create infinite devices and pass the device’s fingerprint reputation.
- Virtual Machines: A virtual machine (VM) is a virtual environment that functions like a computer. It allows you to run an operating system in an app window on your desktop. VMs can simulate a different operating system for development purposes or run a few machines on a single physical machine in cloud computing. This is yet another way fraudsters can create infinite devices and pass the device’s fingerprint reputation or detection measures for device action and velocity.
Especially as generative AI and other tools enable even novice fraudsters to develop sophisticated attacks, security and fraud teams should prepare themselves to defend against these advanced attacks with not only robust device fingerprints, but multiple methods of detection.
How Transmit Security creates robust device fingerprints for fraud detection
To provide a higher level of assurance for trusted devices and known malicious ones, Transmit Security’s Detection and Response Services deploy automatic updates to our device fingerprinting capabilities and balance the tradeoff between consistency and uniqueness in device fingerprints by leveraging large datasets and a wide range of device telemetry, such as device model and distribution, as shown below, as well as unique device properties.
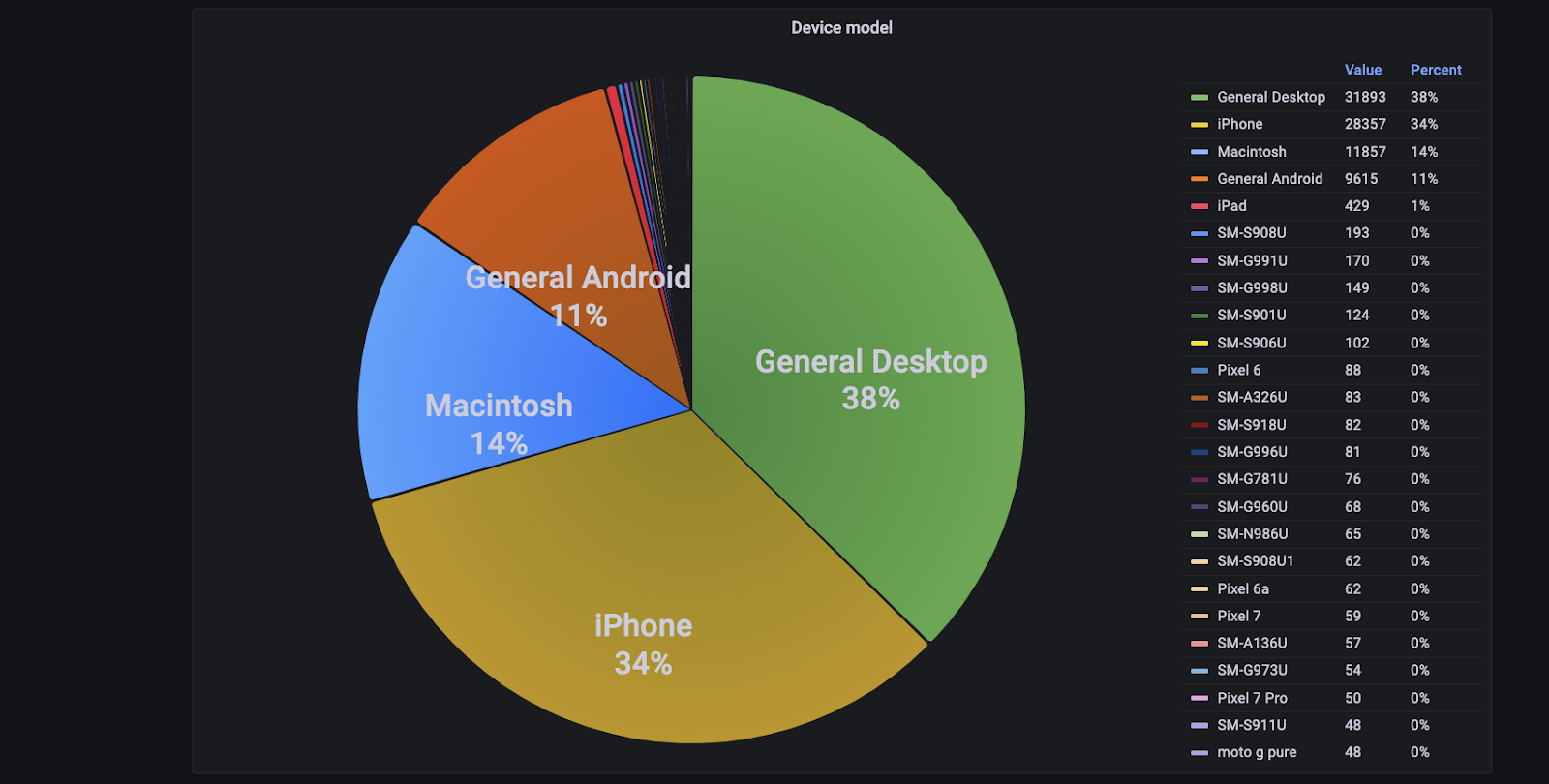
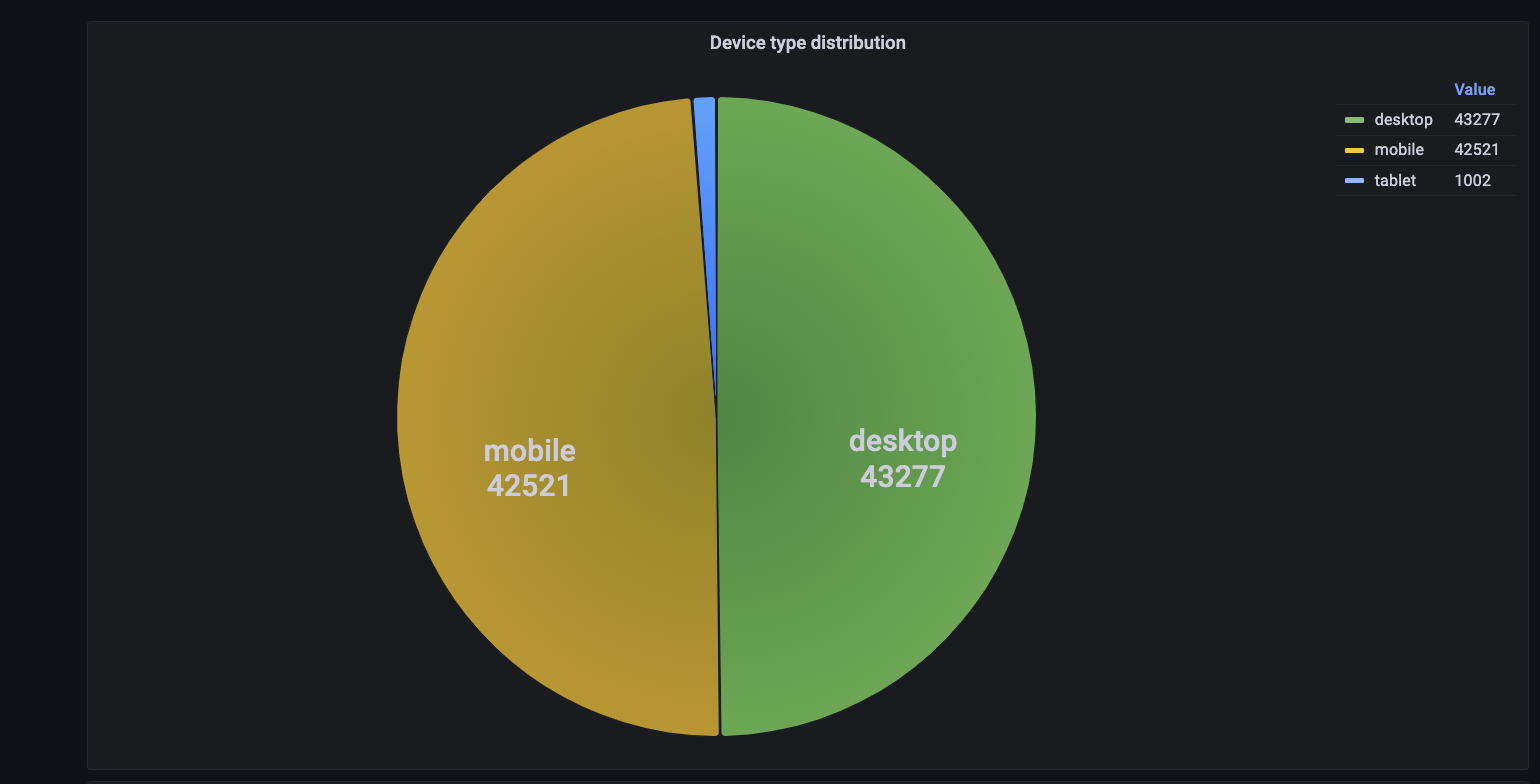
In addition, our device fingerprinting capabilities benefit from our in-house Security Research Labs, where our data scientists constantly research changes in JavaScript engines that impact fingerprinting accuracy, use applied data science to continually improve our true acceptance and true rejection rates and create tools that can be used for detecting anomalies in raw data.
Using these techniques, Transmit Security is able to deliver a 97% true acceptance rate and a 99.7% true rejection rate for device fingerprints — a significantly higher level of assurance than competing solutions.
Conclusion
While device fingerprinting is an effective way to detect and prevent fraud, it’s not a silver bullet. The best strategy is to combine device fingerprint capabilities with other data points such as behavioral biometrics, network reputation, and app activity using advanced AI to get full coverage from advanced types of fraud, which is precisely what Transmit Security Detection and Response is built for.
To learn first hand how to leverage Detection and Response to improve fraud detection, check out our Attack Simulator, which lets you test how automated attacks with evasive properties can be detected on our platform.
Or, to learn about the results businesses can achieve with Detection and Response, read our case study from a leading bank that leveraged our services to detect 10x as many fraud cases as three legacy vendors combined and gain a 1300% return on investment through fraud reduction and vendor consolidation.