Criminals engage in a variety of illicit activities, such as drug sales, illegal arms trade, and human trafficking, often reaping substantial profits. However, to capitalize on these gains, they need to integrate their earnings into legitimate financial systems. This is where the use of money mules comes into play. Detecting and mitigating the presence of mule accounts is imperative for businesses looking to safeguard their financial ecosystem.
Understanding money mules: identifying red flags
Money mules serve as intermediaries for criminal organizations, facilitating their access to legitimate financial services. They fall into three distinct categories:
- Unwilling Participants: Individuals seeking work opportunities who are manipulated into granting criminals access to their bank accounts.
- Willing Participants: Individuals who knowingly assist criminal enterprises, driven by the promise of personal gain.
- Directly Connected Mules: Individuals closely affiliated with the criminal organization, typically opening bank accounts (often under synthetic IDs) expressly for laundering illicit funds.
Money mule process: recruitment to circulation
Often, individuals fall prey to money mule scams, responding to enticing job listings or promises of easy money for minimal effort. Once engaged, the criminal instructs them to move money on behalf of their “employer,” encompassing various forms such as cash, cryptocurrency, gift cards, direct deposits, or receiving physical goods at their address. The participant, now a money mule, is promised compensation for their services.
Let’s delve into the step-by-step process, from the recruitment of an unsuspecting participant to the intricate maneuvers of circulating funds illicitly through their accounts.
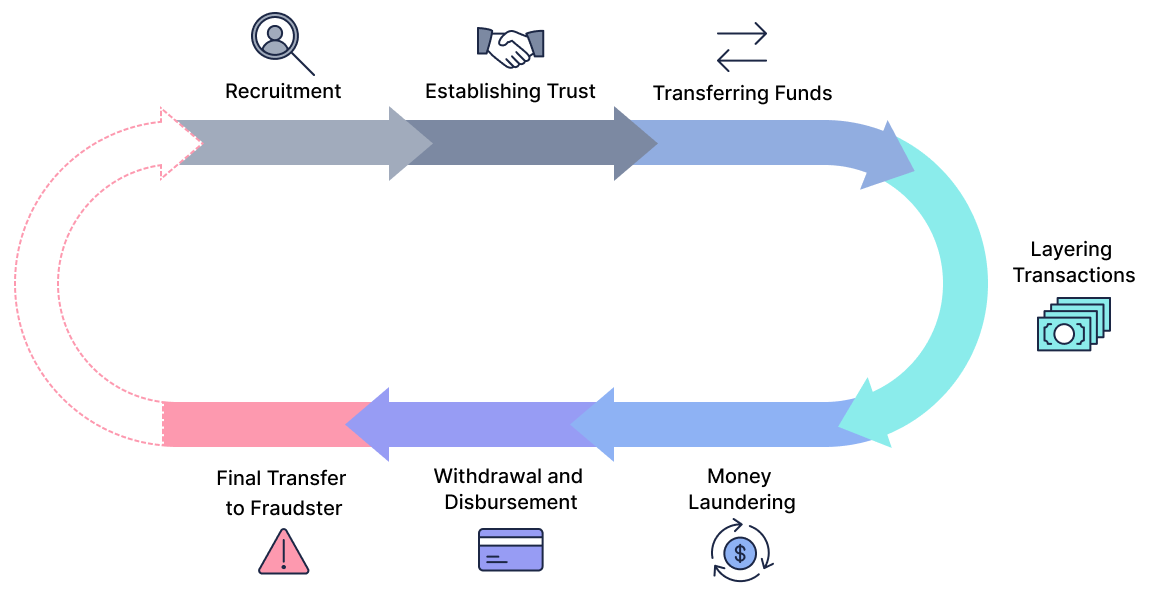
- Recruitment: The fraudster recruits an individual, often through online platforms, social media, or even direct messaging. They may pose as a legitimate employer, offering a job opportunity that involves simple financial transactions.
- Establishing Trust: The fraudster builds trust with the recruited individual, convincing them that the job is legitimate. They may provide convincing documentation or present themselves as a legitimate business.
- Transferring Funds: The fraudster provides specific instructions to the mule on how to receive and transfer funds. This might involve receiving money into their bank account and then forwarding it to other accounts or individuals.
- Layering Transactions: The process often involves multiple transactions to create a complex web of movement, making it harder for authorities to trace the source of the funds.
- Money Laundering: The ultimate goal of this scheme is to “clean” the funds, making it difficult to connect them to their illicit origin. This can involve various techniques to make the money appear legitimate.
- Withdrawal and Disbursement: In some cases, the mule may be instructed to withdraw cash from an ATM or use other methods to disburse the funds.
- Final Transfer to Fraudster: After a series of transactions, the funds may ultimately end up back in the hands of the fraudster, often in a way that makes it difficult to directly link the mule to the initial theft.
Behavioral analytics: an indispensable shield
The global upheaval caused by the COVID-19 pandemic has led to a surge in individuals in the APAC region falling into poverty. Criminals seized this opportunity to recruit more people as money mules, with young individuals in their teens and twenties being particularly susceptible.
The consequences of successful money mule operations can be severe for APAC banks. Recent data reveals that collective fines paid by APAC banks for non-compliance with anti-money laundering (AML) requirements surpassed $10.6 billion USD.
Criminals turn to money mules because they believe banks perceive them as trustworthy intermediaries. However, recent technological advancements, notably in behavioral analytics, empower banks to track and identify criminals involved in money laundering schemes, enabling them to detect such activity earlier and respond more swiftly.
Unraveling mule scams: the key to detection
Behavioral analytics is the linchpin in combating money laundering. It enables banks to comprehend their customers’ typical behaviors through user profiling and flag deviations that may indicate mule account activity using event intelligence and behavioral biometrics.
These solutions have already proven their effectiveness in thwarting account takeover (ATO) fraud attacks. By scrutinizing parameters like touchscreen interactions, keystroke activity, mouse movements, and device orientation and handling, behavioral biometrics are used in tandem with event intelligence from other detection methods, such as device fingerprinting, geolocation analysis and IP analysis, to build a profile of each user’s behavior. These user profiles of historical behavior are then compared to each new request from the user to authenticate whether the person behind the screen is indeed the legitimate customer, effectively discerning them from potential criminal actors.
In essence, behavioral analytics furnishes financial institutions with a suite of seamless security controls, accelerating and refining the fraud detection process for greater precision. Furthermore, the application of behavioral analytics extends beyond fraud prevention, providing a new dimension for banks to identify and counteract money mules attempting to exploit their services for illicit gain.
Four distinct benefits of behavioral analytics for mule account detection
Delving deeper into behavioral analytics applications, financial institutions are leveraging this technology not only as a defense mechanism against unauthorized access, but as a proactive tool to thwart the entire chain of fraudulent financial activity. This translates to not only identifying atypical transaction behaviors indicating a compromised account, but also pinpointing and mitigating the signs of mule account utilization and creation.
Specific applications examples include:
- Flagging abnormal User Behavior: Identifies uncertainty or unusual behavior during transfers.
- High-Risk Transaction Identification: Detects engagement with unfamiliar accounts, signaling potential mule activity.
- Preventing Fraudulent Onboarding: Flags suspicious behavior during registration and account creation, thwarting criminals from opening accounts for money laundering.
- Unraveling Criminal Networks: Identifies individuals linked to criminal operations, enabling banks to trace other mule accounts under their control and report such activity to authorities.
Layered security: combating fraud rings
Detecting mule accounts requires a multi-faceted approach. In addition to behavioral analytics, businesses must employ sophisticated fraud ring detection techniques. This involves connecting the dots across various keys such as device information, device fingerprints, session behavior, and IP addresses. By identifying patterns indicative of organized criminal activity, businesses can effectively thwart money mule operations.0
Verifying users through secure identity proofing
Secure identity proofing, notably identity verification and data validation, effectively verifies users during pivotal moments like account creation and when suspicious behavior is observed. Identity verification is an active validation mechanism, where the user provides a selfie and ID for analysis, thereby ensuring a high level of assurance in its results. Data validation is a passive validation system, seamlessly verifying emails, physical addresses and phone numbers in the background without needing user intervention.
Both these systems, in isolation or in conjunction, have proven to be potent in detecting fraudulent activities during the account opening phase. Moreover, their importance becomes even more pronounced during high-risk scenarios. For instance, utilizing data validation to scrutinize the reputation of phone numbers can be invaluable when there’s a change in two-factor authentication settings—a common red flag indicating a potentially compromised or sold account.
To combat money mules, employing identity proofing can aid in identifying if an account being created is synthetic or associated with a criminal entity. Concurrently, data validation scrutinizes the legitimacy of the data provided during account creation or changes in settings. For instance, analyzing the reputation of phone numbers during two-factor authentication (2FA) setup helps in identifying potential risks, ensuring the account hasn’t been traded off and exploited for fraudulent activities, thus strengthening the measures in place to detect and counter money mules effectively.
Seamless implementation for enhanced security
Just like data validation, behavioral analytics-based AI technology operates silently in the background, leveraging machine learning risk models to identify unusual behavior indicative of suspicious activity. This ensures that customers can proceed with their business uninterrupted, while banks have the confidence that only legitimate customers are using their services.
Criminals rely on everyday individuals with legitimate bank accounts to act as money mules in their schemes. Understanding the typical behavior of these account holders is key to uncovering illegal activity.
As technology evolves and fraud tactics become increasingly sophisticated, behavioral analytics stands as a central pillar in the battle against financial crime. It provides a comprehensive suite of security measures that not only streamline fraud detection but also empower banks to identify and counteract money mules seeking to exploit their services.
By harnessing the power of advanced machine learning models, Transmit Security equips businesses with the tools needed to effectively detect and combat money mule activity, fortifying their operations against the evolving tactics of criminals.