Identity verification systems increasingly use AI to provide remote, online assurance of end users’ identities by matching users’ photos with their IDs. But when these solutions are implemented in a way that is unfair or biased, businesses run the risk of harming — rather than protecting — their users, and can suffer significant losses, regulatory consequences or brand damage as a result.
This kind of systematic discrimination by decision-making algorithms is known as AI bias, a phenomenon that is common in identity verification systems. And because these algorithms are designed to scale and used by financial institutions, healthcare providers, mobile operators and other businesses that provide critical services, it’s all the more important to ensure they are implementing systems that work for everyone.
This blog post is designed to help decision-makers understand AI bias, what causes AI bias in identity proofing systems and how Transmit Security mitigates bias in its Identity Verification Services.
Understanding AI bias
All AI systems are subject to bias — the problem occurs when that bias predisposes a model toward unfair or inaccurate decisions for a specific demographic, such as a given age group, race or gender. This happens because AI systems learn to make decisions based on training data, and the quality of that data may be impacted by historical or social inequities or bias in the humans who select and analyze the data.
It’s an example of the garbage in, garbage out adage in machine learning: biased data results in biased decision-making, and the end result is a model with significantly higher error rates among individuals underrepresented groups.
And because AI models are often black boxes that do not explain what factors led to their decisions, these biases can go unnoticed and unchecked for long periods of time, amplifying and codifying existing policies that discriminate against minority populations.
What causes AI bias in identity verification systems?
Identity verification is typically a two-step process. First, users take a photo of their ID, which is used to extract data and check for signs of forgery or tampering, then they provide a selfie that is analyzed to ensure the user is present and the same person represented in the ID.
To compare these images, parameters such as facial symmetry, skin tone and texture, the measurements of facial features and the shape of different parts of the face are extracted from the ID photo and used to create a digital signature, which is compared to that of the user’s selfie.
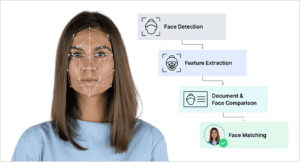
However, some features may produce more accurate results than others, and an attempt to analyze too many data points can make it harder to detect patterns amid the noise. In addition, a lack of sufficient training data for underrepresented groups could cause the model to ignore or give less importance to features that are needed to accurately distinguish faces in these groups. As a result, data scientists must ensure the model is focusing on the features that are most likely to produce effective and unbiased results — a process known as feature selection.
Historical and institutional biases can also skew identity verification results against certain demographics. For example, digital camera sensors are often calibrated against lightness to define facial images, making them less effective with darker-skinned subjects. This is amplified when facial detection algorithms distinguish faces by capturing the intensity of contrast between features such as the eyes, cheek and nose.
How does Transmit Security mitigate the risk of AI bias?
Transmit Security holds itself to the highest standards in developing equitable AI systems, taking steps to monitor and mitigate bias across the AI lifecycle — from the model selection through deployment and production. Some of the measures we use include:
- Deep learning architectures: We use a state-of-the-art multi-task convolutional neural network, which uses three cascaded models to improve the detection of faces and selection of facial landmarks used for analysis.
- Diverse datasets: Our models train on over 400,000 images including multiple public datasets designed to tackle bias, such as the Pilot Parliaments Benchmark, with labeled data from balanced and diverse user groups.
- Data sampling: We use data sampling techniques to help balance datasets, such as stratified sampling, which divides populations into subgroups of users within the same demographic and chooses a proportional number of samples to train the model on, ensuring that groups with fewer data samples are given proper consideration.
- Monitoring and validation: After testing the model, we break down the number of false positives and negatives for different races, genders and age groups and evaluate results against benchmark datasets. This monitoring is continued throughout the model’s lifecycle so we can quickly detect any bias introduced and apply correction techniques as needed.
- Model explainability: We provide transparency into the reasons for each deny or challenge recommendation with precise, human-readable descriptions that help distinguish between failures that could be due to AI bias in facial matching from those that are due to user error or other risk signals.
Conclusion
Online access to critical services is becoming a necessity for users, making secure digital onboarding with ID checks more important than ever. But while AI holds the key to scaling these crucial services for enterprise use, vendors and businesses alike have a responsibility to ensure that these decisions are fair and impartial — ensuring that they do not simultaneously scale existing biases and discrimination.
Learn more about how our Identity Verification Services enable secure onboarding for users in this blog post, or contact Sales to set up a free personalized demo.